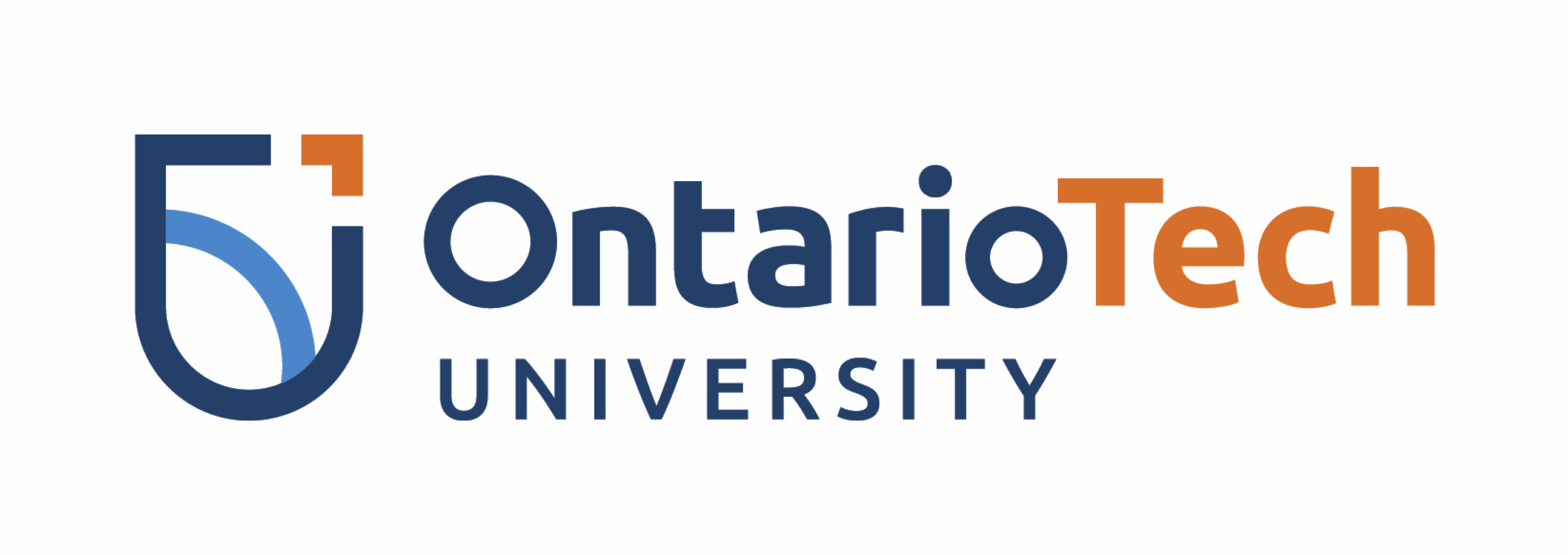
Diffusion Models¶
Faisal Qureshi
faisal.qureshi@ontariotechu.ca
http://www.vclab.ca
Lesson Plan¶
- Diffusion Models
What are Diffusion Models?¶
- Probabilistic generative models that learn to transform noise into structured data.
- Inspired by physical diffusion processes.
Diffusion Process¶
Forward Diffusion (Adding Noise)¶
- Gradually adds Gaussian noise to data over T timesteps:
$$
q(x_t | x_{t-1}) = \mathcal{N}(x_t; \sqrt{1-\beta_t} x_{t-1}, \beta_t I)
$$
- $ \beta_t $ is a small noise variance schedule.
Reverse Process (Denoising)¶
- Learns to denoise step-by-step to generate realistic MNIST digits: $$ p(x_{t-1} | x_t) = \mathcal{N}(x_{t-1}; \mu_\theta(x_t, t), \Sigma_\theta(x_t, t)) $$
Training Objective¶
- Train a neural network to predict the noise ( \epsilon ): $$ L = \mathbb{E}_{q(x_t | x_0)} \left[ || \epsilon - \epsilon_\theta(x_t, t) ||^2 \right] $$
Implementation using PyTorch¶
In [1]:
import torch
import torch.nn as nn
import torch.optim as optim
import torchvision.datasets as datasets
import torchvision.transforms as transforms
from torch.utils.data import DataLoader
Model¶
In [2]:
# Define Diffusion Model
class DiffusionModel(nn.Module):
def __init__(self):
super().__init__()
self.net = nn.Sequential(
nn.Linear(784, 512),
nn.ReLU(),
nn.Linear(512, 784)
)
def forward(self, x):
return self.net(x)
model = DiffusionModel()
optimizer = optim.Adam(model.parameters(), lr=1e-3)
criterion = nn.MSELoss()
Training¶
In [3]:
# Load MNIST dataset
transform = transforms.Compose([transforms.ToTensor(), transforms.Lambda(lambda x: x.view(-1))])
dataset = datasets.MNIST(root='../datasets/common', train=True, transform=transform, download=True)
dataloader = DataLoader(dataset, batch_size=64, shuffle=True)
for epoch in range(10):
for batch in dataloader:
x, _ = batch
optimizer.zero_grad()
noise = torch.randn_like(x)
x_noisy = x + noise # Simulating forward process
predicted_noise = model(x_noisy)
loss = criterion(predicted_noise, noise)
loss.backward()
optimizer.step()
print(f"Epoch {epoch+1}, Loss: {loss.item():.4f}")
Epoch 1, Loss: 0.4003
Epoch 2, Loss: 0.3891
Epoch 3, Loss: 0.3929
Epoch 4, Loss: 0.3875
Epoch 5, Loss: 0.3789
Epoch 6, Loss: 0.3866
Epoch 7, Loss: 0.3831
Epoch 8, Loss: 0.3808
Epoch 9, Loss: 0.3782
Epoch 10, Loss: 0.3786
Use¶
In [4]:
import matplotlib.pyplot as plt
def visualize(model, dataloader):
model.eval()
for batch in dataloader:
x, _ = batch
noise = torch.randn_like(x)
x_noisy = x + noise
with torch.no_grad():
x_denoised = model(x_noisy)
fig, axes = plt.subplots(2, 10, figsize=(10, 2))
for i in range(10):
axes[0, i].imshow(x_noisy[i].view(28, 28), cmap='gray')
axes[1, i].imshow(x_denoised[i].view(28, 28), cmap='gray')
plt.show()
break
visualize(model, dataloader)
Optimizations for MNIST Generation¶
- Use a U-Net Architecture for improved denoising.
- Adjust Noise Schedule ($ \beta_t $) for better results.
- Use DDIM (Denoising Diffusion Implicit Models) for faster generation.
Comparing Generative Models for MNIST¶
Model Type | Strengths | Weaknesses |
---|---|---|
GANs | Fast, sharp images | Mode collapse, unstable training |
VAEs | Good latent space, stable | Blurry samples |
Diffusion | Stable, diverse samples | Slow generation |
Conclusions for MNIST¶
- Diffusion models can effectively generate MNIST digits.
- Robust training with fewer artifacts than GANs.
- Future improvements focus on efficiency and scalability.
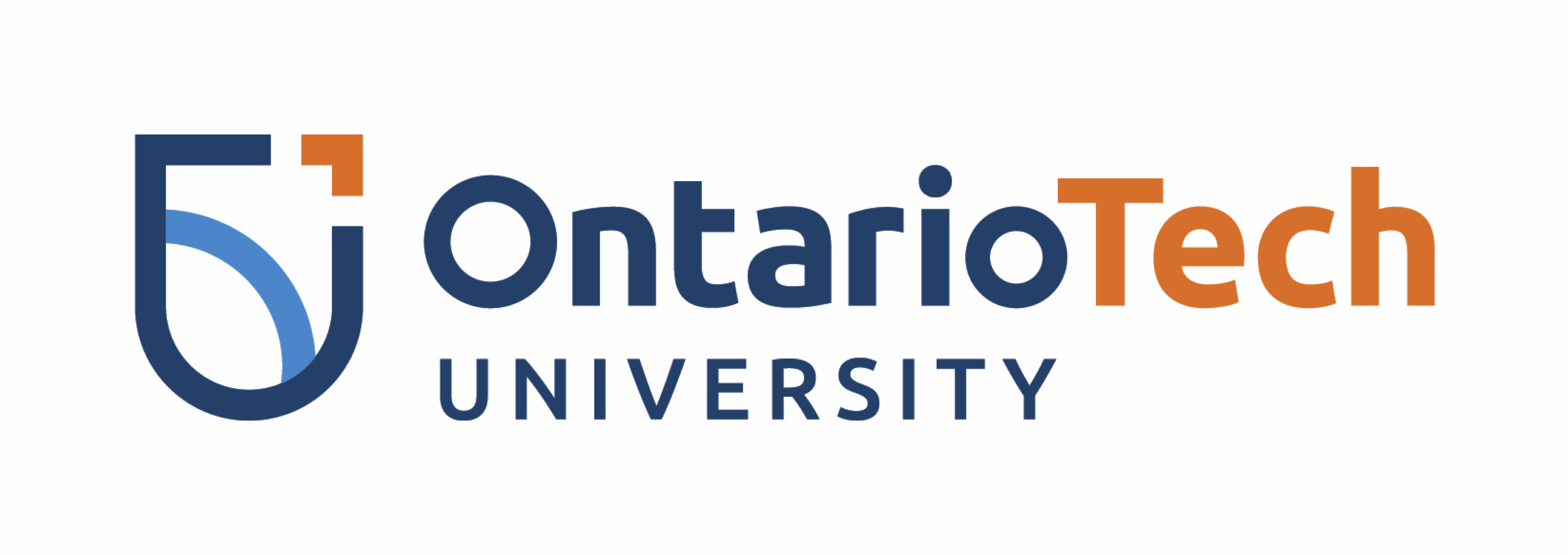