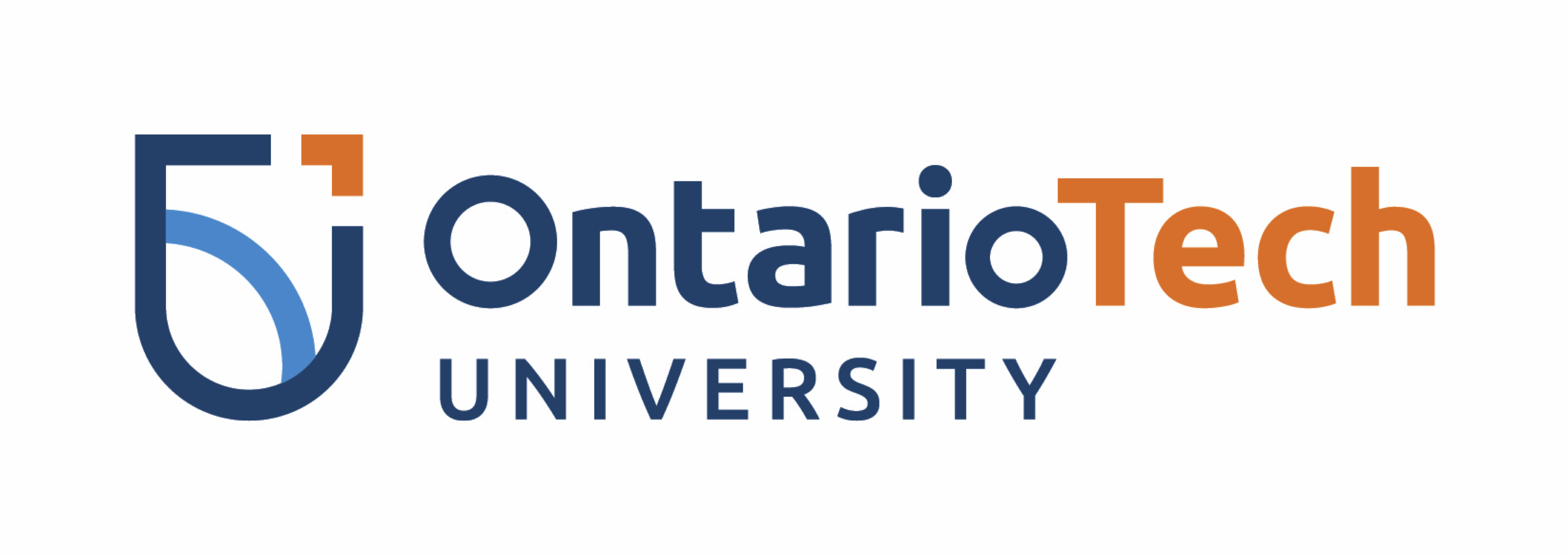
CIFAR10 Image Classification¶
Faisal Qureshi
Professor
Faculty of Science
Ontario Tech University
Oshawa ON Canada
http://vclab.science.ontariotechu.ca
Copyright information¶
© Faisal Qureshi
Acknowledgements¶
Taken from https://pytorch.org/tutorials/beginner/blitz/cifar10_tutorial.html
License¶
This work is licensed under a Creative Commons Attribution-NonCommercial 4.0 International License.
Introduction¶
The purpose of this lab is to study image classification. Specifically, we will attempt to classify CIFAR10 datasets using a Multilayer Perceptron (MLP). We will use PyTorch to train this model.
The code provided below trains and evaluates a $10$-way linear classifier, i.e., it takes all the pixels of a CIFAR10 image (across three channels)--$32 \times 32 \times 3$--and fits $10$ lines, one for each class, to it. The figure shown below capture this model.
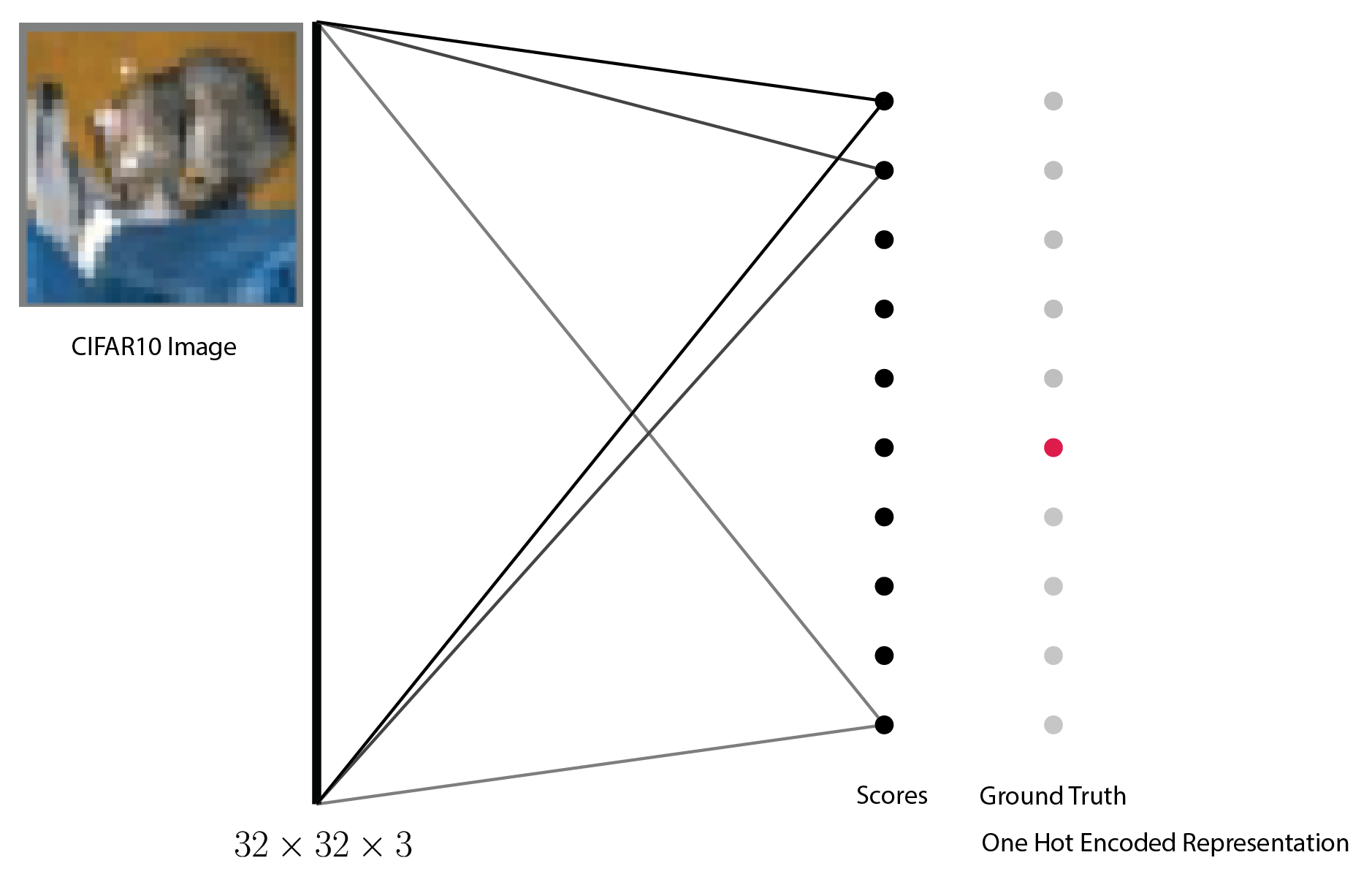
Tasks¶
The goal of the lab is three-folds.
- Understand how PyTorch is being used to setup a model.
- Capture the performance of this linear model on the problem of image classification.
- Convert the linear model to a Multi-layer Perceptron Model (MLP) and compare the performance of the two models.
- I suggest that you start with a model with a single hidden layer. The hidden layer will have an activation function, say ReLU. The output layer will take the hidden layer and outputs $10$ values.
- (Optional) what other changes can you make to the image to improve classification scores.
Sample Code¶
import torch
import torchvision
import torchvision.transforms as transforms
# GPU usage
# device = torch.device('cuda:0' if torch.cuda.is_available() else 'cpu')
# Assuming that we are on a CUDA machine, this should print a CUDA device:
print(device)
cuda:0
transform = transforms.Compose(
[transforms.ToTensor(),
transforms.Normalize((0.5, 0.5, 0.5), (0.5, 0.5, 0.5))])
batch_size = 4
trainset = torchvision.datasets.CIFAR10(root='./data', train=True,
download=True, transform=transform)
trainloader = torch.utils.data.DataLoader(trainset, batch_size=batch_size,
shuffle=True, num_workers=2)
Files already downloaded and verified
testset = torchvision.datasets.CIFAR10(root='./data', train=False,
download=True, transform=transform)
testloader = torch.utils.data.DataLoader(testset, batch_size=batch_size,
shuffle=False, num_workers=2)
Files already downloaded and verified
classes = ('plane', 'car', 'bird', 'cat',
'deer', 'dog', 'frog', 'horse', 'ship', 'truck')
import matplotlib.pyplot as plt
import numpy as np
def imshow(img):
img = img / 2 + 0.5 # unnormalize
npimg = img.numpy()
plt.imshow(np.transpose(npimg, (1, 2, 0)))
plt.show()
dataiter = iter(trainloader)
images, labels = next(dataiter)
# show images
imshow(torchvision.utils.make_grid(images))
# print labels
print(' '.join(f'{classes[labels[j]]:5s}' for j in range(batch_size)))
dog car ship dog
10-way linear model¶
import torch.nn as nn
import torch.nn.functional as F
class ALinearModel(nn.Module):
def __init__(self):
super().__init__()
self.fc = nn.Linear(32*32*3, 10)
def forward(self, x):
x = torch.flatten(x, 1) # flatten all dimensions except batch
x = self.fc(x)
return x
linear_model = ALinearModel()
MLP model (TO DO)¶
class MyMLP(nn.Module):
def __init__(self):
super().__init__()
# TODO
def forward(self, x):
# TODO
return torch.zeros((1,10))
Training¶
import torch.optim as optim
criterion = nn.CrossEntropyLoss()
optimizer = optim.SGD(net.parameters(), lr=0.001, momentum=0.9)
for epoch in range(2): # loop over the dataset multiple times
running_loss = 0.0
for i, data in enumerate(trainloader, 0):
# get the inputs; data is a list of [inputs, labels]
inputs, labels = data[0], data[1]
# zero the parameter gradients
optimizer.zero_grad()
# forward + backward + optimize
outputs = linear_model(inputs)
loss = criterion(outputs, labels)
loss.backward()
optimizer.step()
# print statistics
running_loss += loss.item()
if i % 2000 == 1999: # print every 2000 mini-batches
print(f'[{epoch + 1}, {i + 1:5d}] loss: {running_loss / 2000:.3f}')
running_loss = 0.0
print('Finished Training')
[1, 2000] loss: 2.361 [1, 4000] loss: 2.352 [1, 6000] loss: 2.361 [1, 8000] loss: 2.366 [1, 10000] loss: 2.364 [1, 12000] loss: 2.364 [2, 2000] loss: 2.360 [2, 4000] loss: 2.361 [2, 6000] loss: 2.366 [2, 8000] loss: 2.360 [2, 10000] loss: 2.359 [2, 12000] loss: 2.364 Finished Training
Saving Model¶
PATH = './cifar_linear_model.pth'
torch.save(linear_model.state_dict(), PATH)
Inference on a test images¶
dataiter = iter(testloader)
images, labels = next(dataiter)
# print images
imshow(torchvision.utils.make_grid(images))
print('GroundTruth: ', ' '.join(f'{classes[labels[j]]:5s}' for j in range(4)))
GroundTruth: cat ship ship plane
linear_model = ALinearModel()
linear_model.load_state_dict(torch.load(PATH))
<All keys matched successfully>
outputs = linear_model(images)
_, predicted = torch.max(outputs, 1)
print('Predicted: ', ' '.join(f'{classes[predicted[j]]:5s}'
for j in range(4)))
Predicted: deer dog plane ship
Computing accuracy on the entire test set¶
correct = 0
total = 0
# since we're not training, we don't need to calculate the gradients for our outputs
with torch.no_grad():
for data in testloader:
images, labels = data
# calculate outputs by running images through the network
outputs = linear_model(images)
# the class with the highest energy is what we choose as prediction
_, predicted = torch.max(outputs.data, 1)
total += labels.size(0)
correct += (predicted == labels).sum().item()
print(f'Accuracy of the network on the 10000 test images: {100 * correct // total} %')
Accuracy of the network on the 10000 test images: 8 %
Computing class-wise accuracy on the entire test set¶
# prepare to count predictions for each class
correct_pred = {classname: 0 for classname in classes}
total_pred = {classname: 0 for classname in classes}
# again no gradients needed
with torch.no_grad():
for data in testloader:
images, labels = data
outputs = linear_model(images)
_, predictions = torch.max(outputs, 1)
# collect the correct predictions for each class
for label, prediction in zip(labels, predictions):
if label == prediction:
correct_pred[classes[label]] += 1
total_pred[classes[label]] += 1
# print accuracy for each class
for classname, correct_count in correct_pred.items():
accuracy = 100 * float(correct_count) / total_pred[classname]
print(f'Accuracy for class: {classname:5s} is {accuracy:.1f} %')
Accuracy for class: plane is 26.2 % Accuracy for class: car is 4.4 % Accuracy for class: bird is 8.8 % Accuracy for class: cat is 4.8 % Accuracy for class: deer is 4.6 % Accuracy for class: dog is 12.4 % Accuracy for class: frog is 5.1 % Accuracy for class: horse is 5.6 % Accuracy for class: ship is 5.6 % Accuracy for class: truck is 8.3 %
What to submit¶
- Python notebook that implements multilayer perceptron model.
- A report that lists the classification performance of the linear and MLP model.
- (Optional) Python notebook that implements some of the changes that you have made to the model that improves the classification scores.
All work should be submitted to Canvas.
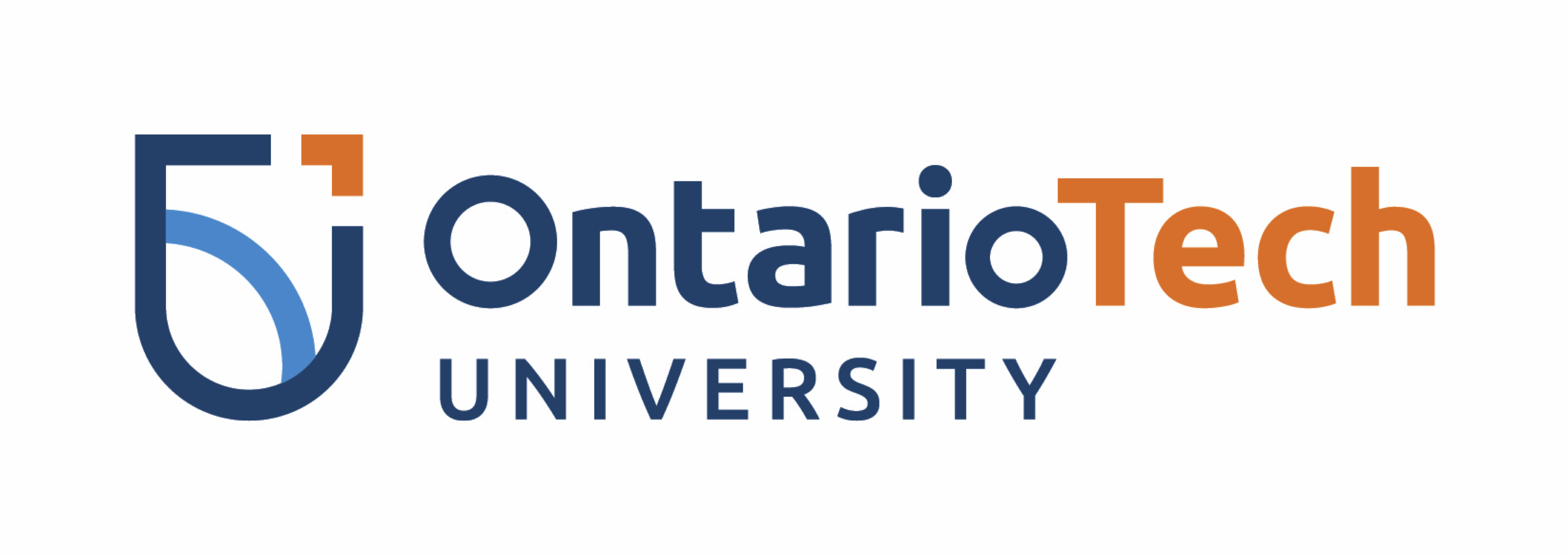